Funding Overview
Our research unit is working on several 3rd party projects funded by the European Union's Horizon2020 and Horizon Europe programs, the German Research Foundation, the German Federal Ministry of Education and Research, and the German Federal Ministry of Economic Affairs and Energy:
Current funding
Modern cancer therapeutics target signalling processes within the cancer cells and the interaction of cancer and immune cells. A comprehensive understanding of these signalling processes is therefore essential to identify drug targets, plan clinical trials, and to select suitable drugs, drug combinations and drug dosages for a specific patient. Yet, most of the available mathematical models capture only a small number of molecular species and pathways, thereby ignoring important crosstalk and feedback loops. Furthermore, these models are usually based on experimental data for cell lines, which behave differently from complex cancer tissues.In INTEGRATE, we will develop computational methods for the full process of data-driven modelling of signalling processes in cancer, ranging from model development to parameterisation all the way to uncertainty analysis. To this end, we will combine methods from the fields of mathematical modelling, machine learning, and signal processing with established approaches in systems biology. The model development will employ natural language processing and an automatic testing framework. For federated model inference, we will develop scalable mini-batch optimisation and marginalisation based uncertainty quantification. To refine models, we will exploit tools from signal processing, such as blind identification of latent variables. We will apply the developed scalable mechanistic modelling approach to integrate large-scale biomedical data for molecular phenotyping studies and clinical trials across sites. This will provide mechanistic models reconciling the available data.
The study will, for the first time, combine mechanistic modelling and machine learning for the integrated analysis of patient-derived omics and phenotypic data. By linking these data sources, INTEGRATE will deepen our understanding of biological signal processing and provide the basis for the development of digital twins.
Funding: Horizon Europe - ERC Consolidator Grant 2023 (grant agreement no. 101126146)
Host-pathogen interactions are complex biological processes that determine the outcome of infections. Many of these interactions are still unknown, especially in the case of novel pathogens, which complicates the development of suitable therapies. At the same time, new diagnostic methods provide us with a wealth of data, where current methods reach their limits in analysis.
EMUNE is therefore developing a modular analysis platform based on new machine learning (ML) concepts to improve the analysis of host-pathogen relationships. Through its integrative structure, EMUNE aims to help bridge the gap between large-scale clinical cohort studies and tissue-level understanding of molecular infection processes. To this end, EMUNE brings together experts in ML, data analysis, and mathematical modeling with clinical and experimental partners in infection research. In various subprojects, new tools for data analysis are developed and the infection dynamics of specific pathogens are investigated.
We are primarily working on the development of new methods for modeling host-virus interactions. Invertible neural networks will be used to improve the scalability of the parameterization of non-linear mixed models, thus making these approaches applicable to large population cohorts. Here, we will focus on accelerating marginalization. Complementarily, to minimize data transfer, we will develop federated inference methods that enable efficient distributed learning of mixed models and thus can easily integrate data from different clinical cohorts.
Funding: Federal Ministry of Education and Research (grant no. 031L0293C)
Partners: University Heidelberg (coordination), Technical University Dresden, Clinical Center of the University of Munich
Webpage: https://emune-dev.github.io/
Public health crises, such as a pandemic, require decisive political action. Governments can take a range of nonpharmaceutical measures of varying intensity, ranging from voluntary social distancing of individuals to mandatory country shutdowns. These non-pharmaceutical measures (NPMs) influence the spread of disease and can prevent overuse of the health care system.
Population-based modeling of the spread of infections has proven indispensable for decision making. However, the insights and predictions provided by existing models are necessarily limited by their resolution and the data on which they are based. In addition, the focus on single pathogens ignores indirect interactions and cross-immunity. To address these issues, the interdisciplinary INSIDe project will develop refined models and data integration strategies that enable integrative spatiotemporal modeling of the spread of multiple diseases at multiple levels of resolution. In contrast to existing approaches, we will also use spatially distributed wastewater samples, which have been shown to serve as early indicators of increases in reported infections and hospitalizations.
The modular open-source platform developed as part of INSIDe will allow integration of different models and modeling approaches for transmission and monitoring. This will facilitate the integration of diverse information ranging from officially reported case rates to sanitation sampling results to social media data, and ultimately enable a better understanding of the impact of testing strategies and NPM.
Funding: Federal Ministry of Education and Research (grant no. 031L0297A)
Partners: Clinical Center of the University of Munich, German Aerospace Center, tandler.com GmbH
Website: inside-consortium.de (coming soon)
Properties of the tumor microenvironment are used as prognostic and predictive biomarkers in oncology. High-content imaging techniques provide highly quantitative information about the tumor microenvironment, including the type and location of cells and their spatial context. However, mechanistic understanding of the evolution of these patterns, as well as their impact on drug response, often remains elusive. While AI methods are well developed in image analysis, mechanistic simulation of interacting cell populations remains computationally intensive given the large number of cell types and processes involved and the spatially constrained tissue architecture.
The overall goal of InterpretTME is to develop interpretable machine learning (ML) methods for the study of complex multi-scale cellular systems, as well as direct application to current questions in cancer immunology. We will work closely with clinical experts as well as with a research group that was one of the first in Germany to establish high-content imaging using state-of-the-art CO detection by indexing (CODEX) multiplexing technology.
All project partners work on the same research campus in Bonn. This allows us to use the developed data-driven model already in the project to answer current research questions. Using systematic analyses of data-driven models, we will investigate the specific role of immune cell subpopulations in different tumor entities for disease progression, as well as the long-term consequences of chemotherapeutic or biological drugs for the tumor microenvironment.
Funding: Federal Ministry of Education and Research (grant no. 031L0308B)
Partners: University Hospital Bonn (coordination)
Webpage: -
Ordinary differential equations (ODE) and differential algebraic equations (DAE) are important tools in life sciences, engineering and many other research areas. They enable integrative analysis of heterogeneous data and thus improved understanding of dynamical systems. However, the simulation and parameterization of ODE and DAE models require tailored and easy-to-use tools. To support the parameterization of ever larger models, scalability and performance are essential requirements. To this end, we have developed the AMICI (Advanced Multi-Language Interface to CVODES and IDAS) research software that enables efficient and scalable simulation of such models. The goal of this project is to make the AMICI research software available for reuse and possible further development beyond its original context, and to establish quality assurance by a community of experts. To achieve this, we will professionalize software development. We will harmonize Python, Matlab, and C++ interfaces and improve the usability, accessibility, and overall quality of the code base. In addition, we will increase the versatility of AMICI by extending support for established standards and implementing common input formats. To support user-centric development, we will build and expand an active group of users through training and developer meetings. To evaluate and improve AMICI, we will use it to study a comprehensive collection of published benchmark problems. This will include a high-dimensional model of signal processing in tumor cells developed in our laboratory. Since AMICI allows us to study forward and backward problems for large-scale biochemical processes, this project - beyond pure software and method development - will contribute to new insights into cellular signal processing and possibly also into processes in other research areas.
Funding: German Research Foundation (project number 443187771)
Partners: -
Webpage: -
The spread of infectious diseases is a multifaceted and dynamic process that depends on the virulence of the pathogen, host immune responses, and human behavior (e.g., adherence to social distancing, mask wearing). The interplay of these factors is not well understood, and interindividual variability (biomedical and socioeconomic) is often ignored. We will examine (1) interindividual variability in host-virus interactions during SARS-CoV-2 infections and (2) decision-making processes of healthy and infected individuals (and their household members) in different economic and social interactions to determine relevant factors for viral transmission versus containment. The project will establish a systems epidemiology approach that takes into account biomedical and (micro-)economic aspects. The Munich-KoCo19 framework will provide virological, immunological/serological, host genetic and clinical characterization data for up to 6000 individuals, as well as information on their behavior and socio-economic profiles. This comprehensive dataset will be analyzed using statistical and machine learning approaches. In addition, we will develop integrative epidemiological-economic models that account for interindividual variability and its influence on decision-making (e.g., whether individuals stay home or go to work, depending on income, savings, or other pre-existing conditions) and ultimately the dynamics of the SARS-CoV-2 epidemic. The integration of multifaceted factors will provide new insights and methods that can be applied to other infections and future epidemics.
Funding: German Research Foundation (project number 458597554)
Partners: Technical University of Munich, Clinical Center of the University of Munich
Webpage: -
To accelerate the development, analysis and simulation of epidemiological models, we developed the research software MEmilio. This modular software toolbox is designed to accommodate the needs of researchers and users. In large parts, the software is written in C++, which is among the fastest and memory-efficient programming languages. Templates are used to allow for various general structures such as models, graphs, or parameter simulations. The aim of this project is to make the research software MEmilio available for reuse and possible further development beyond its original context. Its modular design and automatic creation of Python interfaces incents the implementation of other researchers’ models to create new hybrid models or establish sound comparisons of model performances. To achieve this considerable increase in impact, we will further professionalize the software development. MEmilio already contains lots of unit tests, different continuous integration (CI) pipelines and a review process. We will increase code coverage in C++ and Python to 100% and better distinguish between unit and integration tests. Further quality assurance will be established through a professional community and improvement of the already existing structure. We will adapt MEmilio implementations to external standards to attract new users and developers. We will equip MEmilio with the possibility for (i) standardized model formulation based on the rule-based language Kappa as well as (ii) standardized description of simulation experiments and datasets based on the PEtab format. We will harmonize the interfaces between the different models and programming languages to allow identic execution of all MEmilio models from C++ and Python. General methods like a graph or I/O will be further templated, also using advanced template metaprogramming.We will focus on efficient implementations and scalability to make best use of available resources. Energy-efficient implementations will allow to provide predictions of MEmilio models on time to scientists, politicians, and decision makers. Furthermore, we will increase the versatility of MEmilio by extending the support of community standards and implementing general-purpose input formats. To support user-centered development, we will build an active community of users and developers by offering user training and developer workshops.
Funding: German Research Foundation (project no. 528702961)
Partners: German Aerospece Center
Webpage: -
Vast amount of experimental data for e. g. cells, mouse models and patients will become available in this Collaborative Research Center (CRC) and are collected to assemble a comprehensive picture of metaflammation, culminating in a semantic knowledge, graph-based database, MetaflammDB, made available to the scientists in the CRC. The central project Z01 coordinates central data management (cf. Fig. 1) and contributes to statistical and bioinformatic data analysis, model-based data integration and mechanistic modelling of cellular pathways (cf. Fig. 2) and does support standard data analysis tasks, such as preprocessing, quality control, statistical analysis, and performs advanced data analysis tasks, such as functional and network analysis, deconvolution and data integration. To this end a comprehensive data management infrastructure has been established, supporting reuse, exchange, integration and release of data and metadata following the FAIR principles. To enable the project partners and educate the CRC scientists, training programs will provide an overview of state-of-the-art bioinformatic tools as well as statistical and mechanistic modelling approaches and data and metadata management strategies.
Graphical abstract / figures:
Figure 1: Central management platform and infrastructure for data and metadata
Figure 2: Visual outline of selected contributions in the field of computational biology, ranging from statistical and bioinformatic analysis to detailed mechanistic modelling
Funding: German Research Foundation (SFB 1454, Project number 432325352)
Systems energetics and whole-body metabolism are determined by a multitude of processes, giving rise to an immense degree of complexity. In order to understand these processes and their interconnection in the context of thermogenic adipose tissue, the CRC/TRR will collect a large amount of experimental data for cell line models, mouse models and humans using different experimental approaches.
This project will support this effort by coordinating data management and sharing, establishing the necessary infrastructure and providing training courses. Furthermore, we will contribute to the comprehension of these datasets and the underlying processes by supporting data analysis, including pre-processing, statistical testing, functional analysis and modelling.
The overarching goals of this project are to:
- Establish the central infrastructure for TRR (all subprojects).
- Provide training courses and consulting for the members of TRR (all subprojects).
- Assist with the (reproducible) computational analysis of data (e.g., on brown adipose tissue, thermogenesis and metabolism) collected by the TRR (all subprojects).
- Assist with the sharing and archiving of experimental data on systems energetics and data analysis workflows by TRR in accordance with the FAIR principles (all subprojects).
- Develop a causal modelling pipeline (for cell-cell and organ-organ crosstalk) based on natural language processing to assist with the partners in hypothesis generation.
Funding: German Research Foundation (TRR 33, Project number 450149205)
Website: https://www.trr333.uni-bonn.de/
ORCHESTRA is a three-year international research project aimed at tackling the coronavirus pandemic, led by the University of Verona and involving 26 partners (extending to a wider network of 37 partners) from 15 countries: Argentina, Belgium, Brazil, Congo, France, Gabon, Germany, India, Italy, Luxemburg, Netherlands, Romania, Slovakia, Spain, Venezuela.
Funding: The project is funded by the European Union’s Horizon 2020 research and innovation programme under the ERAvsCORONA Action Plan which was developed jointly by Commission services and national authorities
Website: https://orchestra-cohort.eu/
Previous funding
The relationship between vaccine composition (epitopes, adjuvant) and B- and T-cell response is poorly understood. This makes targeted vaccine development difficult. To help close this knowledge gap, we will develop methods in GENImmune to derive automated simulation models from knowledge databases (such as disease maps) and parameterize them on experimental data. For this purpose, we will combine approaches from the field of artificial intelligence with methods for dynamic modeling. Furthermore, we will develop concepts and tools to interpret the resulting complex models.
The core result of the project will be new generic methods for the analysis of complex infection processes. These methods will be applied in collaboration with GENImmune partners to better understand host-pathogen interactions in COVID-19. We will use the knowledge base generated within the collaborative project to build a simulation model and then train it using publicly available data as well as data generated within the project. This model will then be made available to partners for use in optimizing immunization strategies.
Funding: Federal Ministry of Education and Research (grant no. 031L0292F)
Partners: Biomax Informatics AG (coordination), Technical University Munich, PMCR GmbH, PEPperPRINT GmbH
Webpage: -
Pathological changes in tissue are currently analyzed using quantitative imaging techniques and described using computer models. So far, however, there is no solution to parameterize multicellular models with high throughput and high resolution. In this project, we will implement and validate a user-friendly, open-source software framework for modeling, simulating and parameterizing multicellular processes based on quantitative image data. This software framework can be used in biomedical research in the future. FitMultiCell will combine the strengths of the modelling and simulation tool Morpheus developed at TU Dresden (TUD) with the extended statistical inference tool pyABC developed at Helmholtz Zentrum München (HMGU). FitMultiCell will be applicable on different computer infrastructures and will use Deep Learning.
Funding: Federal Ministry of Education and Research (grant no. 031L0159A)
Partners: Universität Heidelberg, Technische Universität Dresden
Webpage: https://fitmulticell.gitlab.io/
Public policy interventions are critical for the management of pandemics, but predictions about their impact are often flawed as they disregard the behavioral responses by individuals. We will establish an integrative framework combining epidemiological and economic modeling to address this challenge. Using the insights and data generated by the current COVID-19 pandemic, we seek to inform decision making processes in current and future health challenges. In our project E2 Modeling, we combine our expertise in epidemiological and economic models to provide policy-makers with such an integrated assessment of their policy choices.
Funding: Volkswagen Stiftung (reference no. 99 450)
Partners:
Webpage: -
Systems medicine aims at improving disease prevention, diagnosis and therapy by studying the human body as an integrated whole. In contrast to reductionists’ approaches, systems medicine allows to integrate different heterogeneous datasets to achieve a comprehensive understanding of the processes involved. Yet, most research projects are limited to individual pathways and models as well as datasets are rarely reused. Model reuse, extension and integration remain challenges due to improper annotation, non-standard implementation and inaccessibility of the experimental data. This in turn limits collaborative research.
This project aims at igniting and facilitating a process that brings these models and datasets (as well as their developers) closer to each other, that interlinks and connects these models as much as technically possible. To this end, we combine a tailored teaching and networking activity with two focused infrastructure activities that extend the FAIRDOMHub and its network editing and versioning functionality.
Funding: Federal Ministry of Education and Research (grant no. 01ZX1705A)
Partners: Heidelberger Institut für Theoretische Studien, Helmholtz Zentrum München, Technical University of Munich, University of Rostock
Gastric cancer was estimated to be the fifth most common cancer and third leading cause of death from cancer worldwide. Treatment options for gastric cancer patients include surgery, chemotherapy and radiation therapy. However, the overall survival rate remains unsatisfactory and new treatment options are urgently required. Novel drugs targeting members of a family of receptor tyrosine kinases including HER2 and epidermal growth factor receptor (EGFR) have shown mixed success in clinical trials.
In this project a systematic molecular and phenotypic analysis of a panel of gastric cancer cell lines will be performed. From these data we derive mechanistic and statistical models for use in patient stratification. In our subproject, we:
- analyze the relation of molecular and phenotypic properties of gastric cancer cell lines
- reconstruct the signaling pathways of cetuximab and trastuzumab
-
validate the reconstructed signaling pathways and phenotype link based upon MALDI imaging MS patient samples
Partners: Biomax Informatics AG, Helmholtz Zentrum für Infektionsforschung, Helmholtz Zentrum München, Technical University of Munich, Universitätsklinikum Leipzig
The advancement of omics technologies as well as growing amounts of public datasets provide the basis for large-scale mechanistic modeling of biological systems. Mechanistic models help to gain a mechanistic understanding of biological processes instead of providing mere statistical correlations. Such mechanistic models can be used for in-silico experiments to, for example, predict the outcome of a pharmaceutical intervention, which is an important tool for personalized medicine as well as for identifying novel drug targets.
However, the parameterization of such large-scale models is computationally very demanding and currently not yet feasible. Furthermore, improved visualization methods are required to analyze and interpret simulation results and parameter estimates. To this end, CanPathPro aims to generate and integrate novel algorithms and protocols for the large-scale predictive modeling of cancer pathways.
Together with our project partners, we:
- refine hybrid stochastic-deterministic global optimisation methods for maximum likelihood model parameter estimation
- develop methods for the integrated visualisation of the underlying heterogeneous experimental data, model predictions, and statistical measures
- integrate these tools into an easy-to-use platform for the predictive modelling of cancer signalling
- generate hypotheses which will – in close collaboration with experimentalists – be tested in vivo to validate or refine the model
Reaction-diffusion-advection equations are used in many fields of engineering and natural sciences to model spatio-temporal processes. As the parameters of these mathematical models are often unknown, they have to be determined from the available experimental data. Here, the first step is usually to employ optimization to determine the parameter values yielding the best match of the model prediction and the experimental data. In the second step, the uncertainty of these parameter values is analyzed to determine the predictive power of the model. In both steps constraint optimization problems have to be solved. For this reliable optimization algorithms are required which converge robustly. Available methods however fail to meet these reliability requirements for a variety of models. Accordingly, we develop a novel simulation-based optimization and profile likelihood calculation method for reaction-diffusion-advection equations, which exploiting the problem structure evaluate the simulation-based methods to several biological problems, including lateral line formation in zebrafish implement a user-friendly MATLAB toolbox.
Funding: German Research Foundation (project no. 311889786)
Partners: University of Klagenfurt
Webpage: -
The aim of the cooperation project is to develop an integrated system for monitoring and intervention of blood glucose levels in intensive care patients. The intended assistance system is to enable continuous and individual blood glucose monitoring of patients for the first time and is adapted to hospital standards. At the heart of the development is a software unit that uses patient-specific models to predict the optimal insulin supply and regulate the insulin infusion on the basis of blood glucose measurement data and other patient data. The associated measurement sensor system continuously monitors the blood glucose level without blood loss and, together with the insulin infusion unit, is to be addressed via the standard central catheter in intensive care patients. Model-based insulin regulation allows glucose levels to be individually and optimally stabilized and risk situations to be detected at an early stage. As an automated closed-loop system, the monitoring and stabilization of a large number of patients is possible without increased personnel effort, thereby reducing the risk of complications during intensive care treatment and mortality.
Funding: German Federal Ministry for Economic Affairs and Energy (grant no 16KN074236)
Partners: -
Webpage: -
The MoKoCo19 project aims to quantify more precisely the effect of non-pharmacological interventions such as school closures and contact restrictions and thus to improve the prediction of pandemic progression. The core of the project is model-based analyses of the population-based prospective Munich Covid-19 cohort study in combination with data collected by the Robert Koch Institute. The results will be used to help policy makers find the best way out of the current crisis.
Funding: Federal Ministry of Education and Research (grant no 01KI20271)
Partners: -
Webpage: -
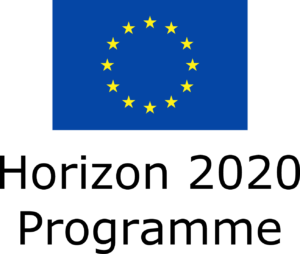
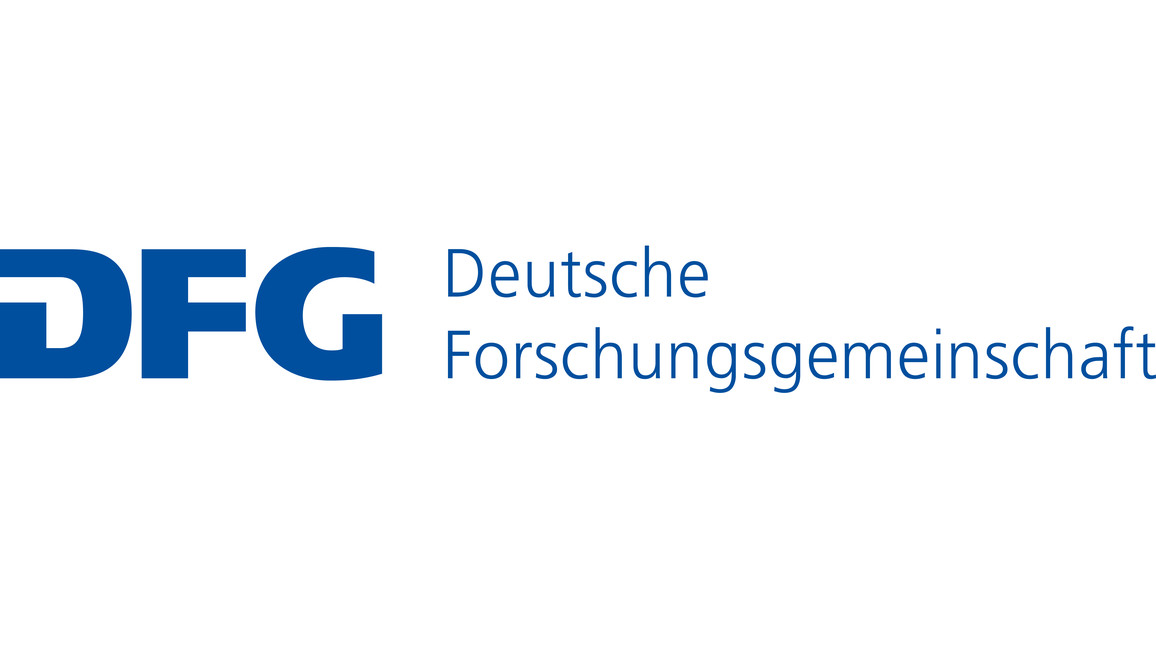
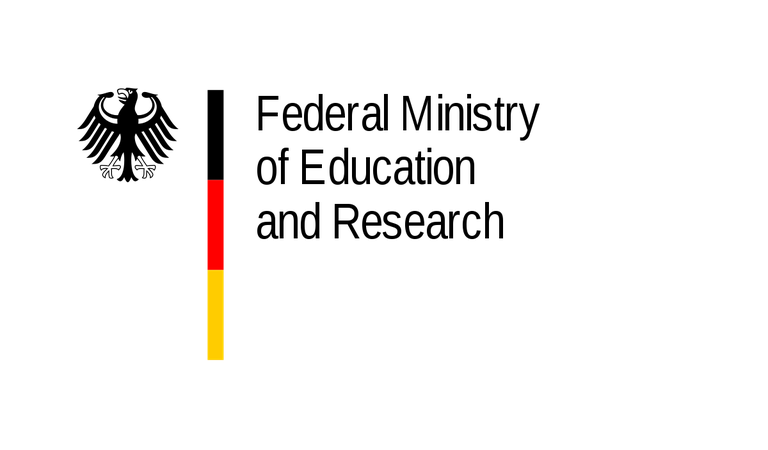
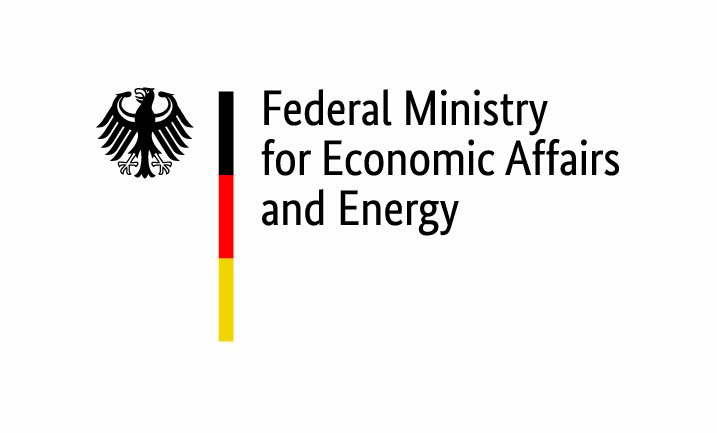